Intelligent intensive care
Background
In intensive care, various devices continuously assess the patient status providing a large amount of data. Medical practitioners must act on such data, most often under time constraints and roughly being able to oversee the huge amounts of information and interconnections. Artificial intelligence (AI) provides the opportunity to tackle this huge amount of data, reducing the burden on the medical staff and allowing not only to react adequately on the current situation but foresee acute events enabling preventive care. Despite a huge potential, many challenges prevent a wide adoption of AI in clinical practice. Algorithms do not yet perform with sufficient precision in many cases. Automated algorithms also need to provide insights on their decision making to facilitate acceptance/trust by clinicians and patients.
?
At DSENS, we work on multiple aspects regarding the usage of physiological time series or other patient data to improve patient monitoring, better identify patients at risk of adverse events and optimize individual care. Projects are ranging from basic to applied research projects involving clinicians and industrial partners.
?
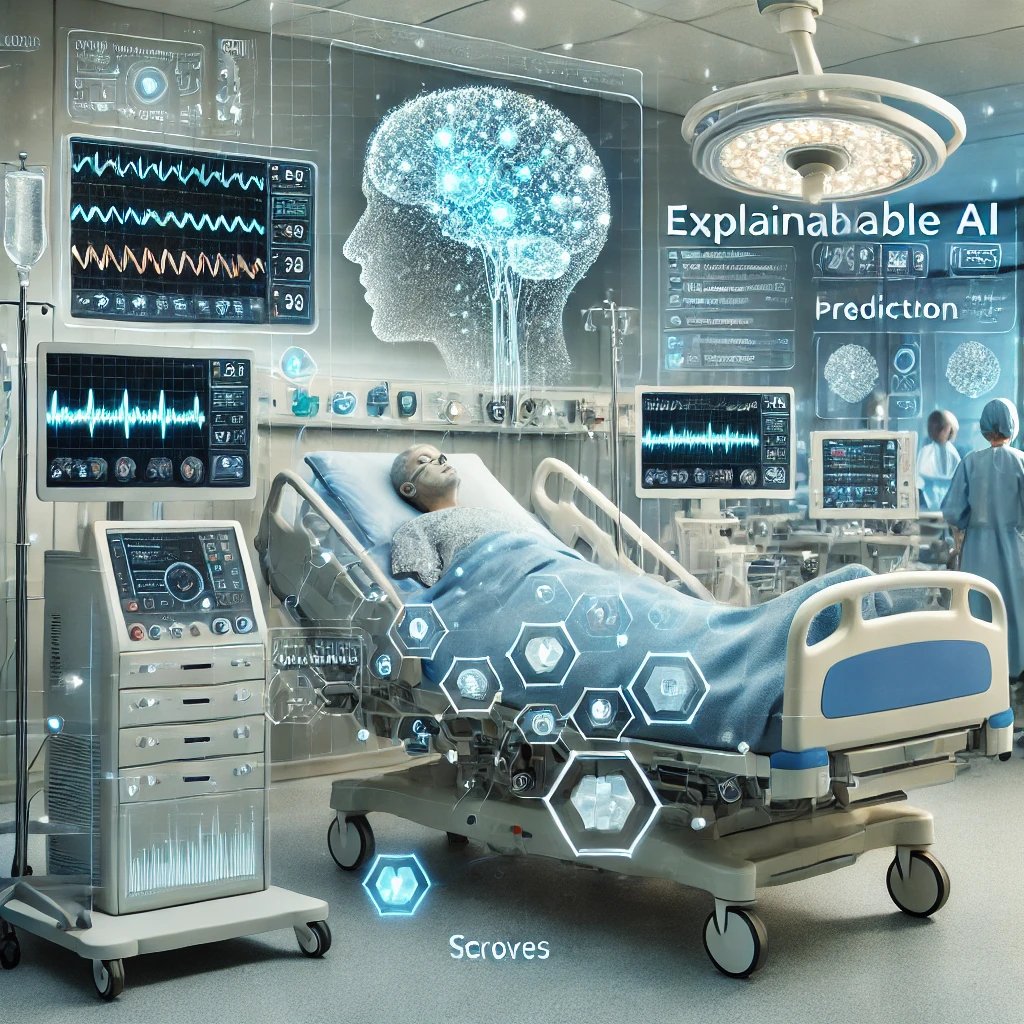
Potential topics (student works)
Possible topics include (but are not limited to) the following aspects
- Analysis or development of algorithms for the non-contact assessment of local perfusion from videos
- Investigations on the usability of hyperspectral reconstruction for vital parameters assessment
- Modelling and simulation of light-tissue interaction by Monte-Carlo simulations
- Conception and implementation of experimental studies invoking fluidic phantoms and healthy volunteers
Note that an individual adjustment is done based on students’ interest and the work to be accomplished (seminar works cover literature research and preparation of the state of the art; project modules, practical modules and theses cover own implementations as well).
?
Further reading
Meyer, A. et al., 2018. Machine learning for real-time prediction of complications in critical care: a retrospective study. The Lancet Respiratory Medicine 6, 905–914.
?
Nasarian, E. et al., 2024. Designing interpretable ML system to enhance trust in healthcare: A systematic review to proposed responsible clinician-AI-collaboration framework. Information Fusion 108, 102412.
?