News
Paper accepted (Oral) at the European Conference on Computer Vision (ECCV) 2024
The paper "A Fair Ranking and New Model for Panoptic Scene Graph Generation" by Julian Lorenz, Alexander Pest, Daniel Kienzle, Katja Ludwig, and Rainer Lienhart has been accepted for ECCV 2024 as an Oral Paper.
The authors discuss significant flaws in commonly used evaluation protocols for Panoptic Scene Graph Generation. They present a solution to this problem and evaluate existing publications based on the new findings.
Finally, a new state-of-the-art architecture for Panoptic Scene Graph Generation is presented.
More information can be found here: https://lorjul.github.io/fair-psgg/
Paper accepted at the eLVM@CVPR 2024 workshop
A paper with the title “Adapting the Segment Anything Model During Usage in Novel Situations” by Robin Sch?n, Julian Lorenz, Katja Ludwig and Rainer Lienhart has been accepted at the workshop for “Efficient Large Vision Models (eLVM)“. The workshop will be held jointly with the CVPR 2024 in Seattle. The paper presents a method for adapting the Segment Anything Model (SAM) during test time without the aid of additional training data. Instead, the method uses information with is generated during usage in order to generate pseudo labels.
Paper for SG2RL@CVPR 2024 accepted
The paper "A Review and Efficient Implementation of Scene Graph Generation Metrics" by Julian Lorenz, Robin Sch?n, Katja Ludwig, and Rainer Lienhart is accepted at the Workshop on Scene Graphs and Graph Representation Learning at CVPR 2024.
?
The authors review existing scene graph generation metrics and provide precise definitions that were lacking in this field. Additionally, they introduce an efficient and easy to use python package that implements all discussed metrics. To improve comparability of new scene graph generation methods, the authors provide a benchmarking service that enables an easy evaluation of scene graph generation models.
?
More information can be found here: https://lorjul.github.io/sgbench/
Open Positions for PhD students
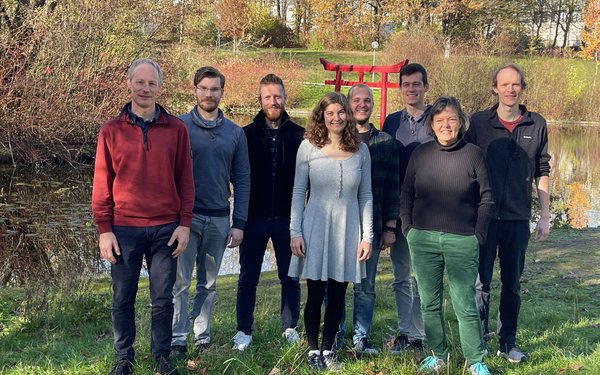
Paper accepted at International Conference on 3D Vision (3DV) 2024
The paper titled "Towards Learning Monocular 3D Object Localization Using the Physical Laws of Motion" by Daniel Kienzle, Julian Lorenz, Katja Ludwig and Rainer Lienhart was accepted to the International Conference on 3D Vision (3DV) 2024. The paper describes a new method for localizing objects in 3D without the need for 3D ground truth. Instead, the method uses knowledge of physical laws to learn the task.
?
See https://kiedani.github.io/3DV2024/ for additional information on the paper.
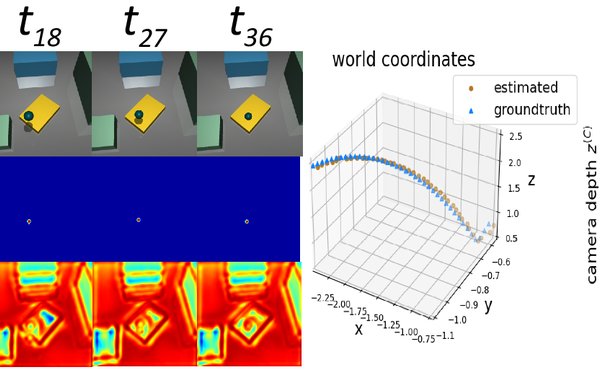
Paper for SG2RL @ ICCV 2023 accepted
The paper “Haystack: A Panoptic Scene Graph Dataset to Evaluate Rare Predicate Classes” by Julian Lorenz, Florian Barthel, Daniel Kienzle, and Rainer Lienhart is accepted at the First ICCV Workshop on Scene Graphs and Graph Representation Learning (SG2RL). The authors present Haystack, a new dataset for scene graph generation that tackles current shortcomings when evaluating with current scene graph datasets. Most notably, Haystack contains rare predicate classes and explicit negative annotations. Only through these properties can rare relationships be reliably evaluated. Based on the design of Haystack, the authors introduce three new scene graph metrics that can be used to gain more detailed insights about the prediction of rare predicate classes.
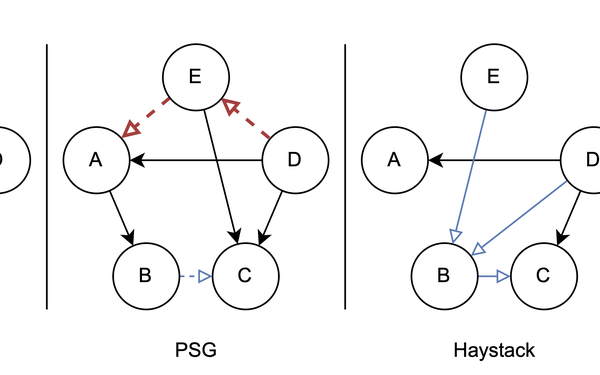
Paper for L3D-IVU @ CVPR 2023 accepted
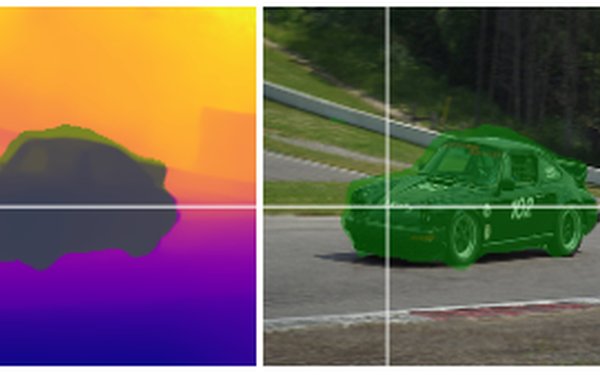
Paper for CVSports @ CVPR 2023 accepted
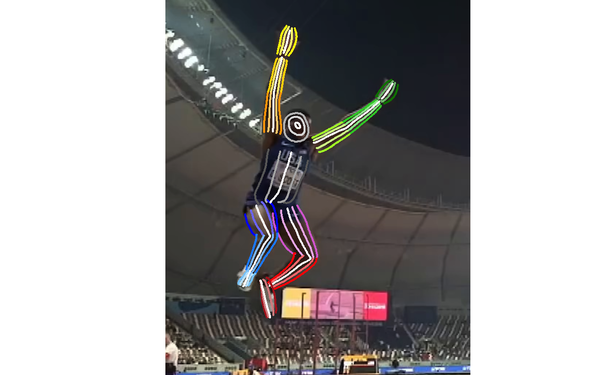
Paper for CV4WS@WACV 2023 accepted
The paper with the title "Detecting Arbitrary Keypoints on Limbs and Skis with Sparse Partly Correct Segmentation Masks" from Katja Ludwig, Daniel Kienzle, Julian Lorenz and Rainer Lienhart is accepted for the workshop Computer Vision for Winter Sports on the?IEEE/CVF Winter Conference on Applications in Computer Vision (WACV) 2023. In this paper, the authors describe how to detect arbitrary keypoints on the limbs and skis of ski jumpers. Only a few, partly correct segmentation masks are necessary in the dataset for the presented method.
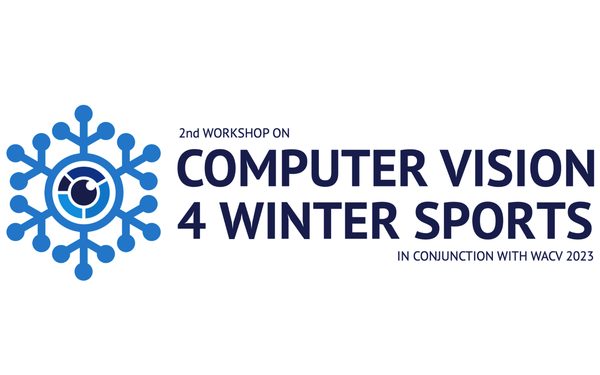
Paper für die BMVC 2022 akzeptiert
Das Paper mit dem Titel "Pseudo-Label Noise Suppression Techniques for Semi-Supervised Semantic Segmentation" von Sebastian Scherer, Robin Sch?n und Rainer Lienhart wurde für die?British Machine Vision Conference (BMVC) 2023?akzeptiert. In diesem Paper beschreiben die Autoren eine Methode die es erm?glich, den Bedarf an gro?en gelabelten Datens?tzen zu verringern, indem nicht gelabelte Daten in das Training einbezogen werden. Als Anwendung verwenden die Autoren die menschlicher Posensch?tzung sowie die semantische Segmentierung, wobei besonderes letzteres interessant ist, da hier die Annotation von Daten ?u?erst zeitaufwendig ist.
First Place in the STOIC Challenge: Prediction of COVID Severity with Convolutional Neural Networks
Daniel Kienzle, Julian Lorenz, Katja Ludwig, Robin Sch?n and Rainer Lienhart from the chair for Machine Learning and Computer Vision achieved the first place in the STOIC challenge. The goal of the challenge was to predict the severe outcome of COVID-19 one month ahead using CT scans. To this end, the researchers employed convolutional neural networks and transfer learning on various tasks. The challenge was organized by Assistance Publique – H?pitaux de Paris, Radboud University Medical Center, and Amazon Web Services.
?